A proactive approach
Predictive maintenance offers big gains in production efficiency. Mike Farish looks at some examples of how these systems have been developed and applied
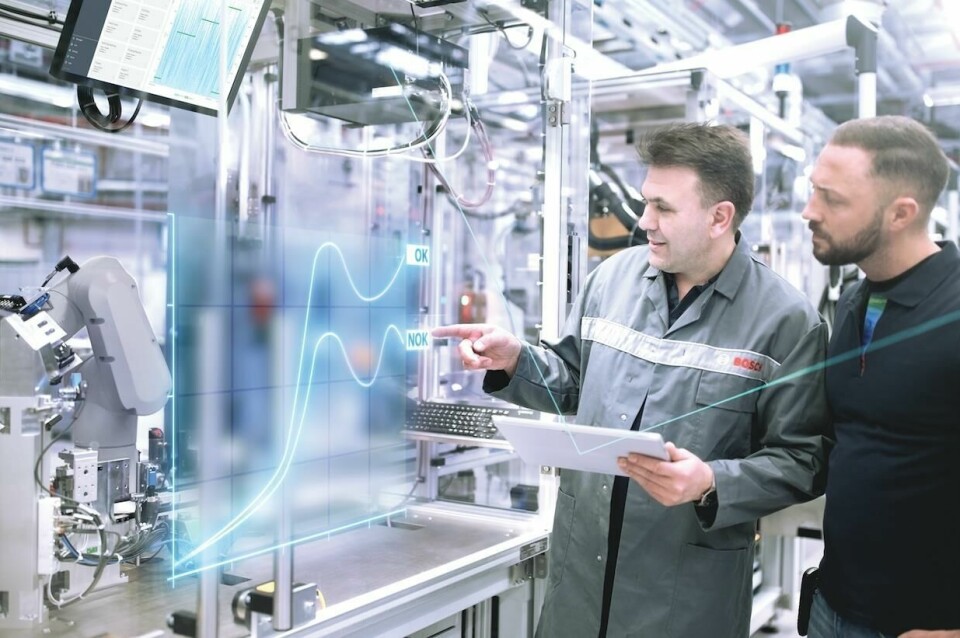
Carmakers are at the forefront of efforts to implement predictive maintenance regimes as they understandably need to avoid potential breakdowns and the ensuing costs. This approach has seen the development and adoption of proactive rather than reactive measures. In turn, suppliers of both production equipment and specific dedicated predictive maintenance software systems are developing increasingly sophisticated technologies to meet that requirement.
An example of this can be seen at the BMW plant at Landshut, Bavaria, which specialises in the production of components to support electromobility and light-weighting. Over the last couple of years, the plant been expanding its use of a software system from Bosch called Nexeed Production Performance Manager, which is described by Daniel Prinzing, senior sales manager with Bosch Connected Industry, as an “Industry 4.0 software solution for systematic production improvement that helps quality and maintenance management staff in decision-making and makes their daily work easier.”
Harmonising production data
Prinzing says that the software comprises individual modules with specific functions including predictive maintenance and real-time process monitoring. He adds that the system “harmonises production data from various sources and can extract customised information from that data and apply production knowledge automatically.” At Landshut the aim was to implement the software across the plant to help achieve live process monitoring independent of location, faster responsiveness within processes and optimisation of maintenance intervals for maximum system availability. The initial application of the software, though, was in a single localised application – in this case on a cockpit production punch press where it was used to monitor punch pressure sensor and slide characteristic values to monitor the condition of the machine, with a key parameter being the run time of the slides.
Right from the start of this ‘starter kit’ phase the Bosch IoT Gateway system has also been used to collect relevant machine data and messages and present them in a user-friendly format. It facilitates, for instance, visualisation of the time required between the retraction and extension of each individual actuator so that it can be provided to the employees in charge. This information makes it possible to detect wear, for example by way of an increase in cycle time or load.
In consequence, with the help of ‘intelligent’ trend analyses employees have better control over the scheduling of maintenance work and minimising downtime. For example, a broken punch knife would previously have resulted in an unscheduled halt in production. But now, detection of increasing slide run times can help track when a knife is becoming dull, so that replacement of the knife can be scheduled for a day when it will have the least impact on production. Predefined rules, which issue automatic warnings when limit values are exceeded, mean that potential disruptions can also be detected early on and eliminated.
“The sensors are optimised for slow moving rotating objects with high sensitivity even in a rough and shaking environment.”Dr Jan Regtmeier, Harting
According to Prinzing, the software can run either locally or in the cloud. “At BMW it is currently operated on the premises,” he states. “A TCP/IP connection between the IoT Gateway and the software is required to send data.” In general, he adds, the data processing capabilities of the software enable staff to implement their own rules in the system: “If they need to trigger events for measured data and machine messages, they can do this themselves without requiring the help of software experts,. The software is browser-based and users can employ PCs, laptops, tablets, smartphones or flat screens on the shopfloor.”
Moreover, as Prinzing also confirms, that initial application at BMW was successful. When asked if it succeeded in decreasing downtime his answer is unequivocal if also limited. “Yes - but we do not have permission to communicate any precise figures,” he states. As a result, the use of the software is being rolled out more widely and is now used for the intricate back-foaming process that is also involved in cockpit production. This enables large amounts of data covering parameters such as pressure, flow rate, temperature and process time that are generated in the control unit but not made permanently available in a higher-level system, to be accessed for process optimisation and to make predictions, for instance regarding wear.
Now individual foaming processes with assignment of variants, tools, mould carriers or segments can be compared directly across any time intervals, making it possible to establish correlations between component quality and machine, process sequence, variant or batch. “
Using i4.0 data and AI algorithms
Senseye, based in Southampton, UK, is a relative newcomer to the provision of predictive maintenance capabilities and “just getting out of the start-up phase” according to CEO Dr Simon Kampa. The company was formally set up some four-and-a-half years ago with the aim of bringing highly specialised predictive maintenance expertise, derived from experience of the aerospace industry, to a wider industrial context with the automotive industry a prime target area.
The company offers a mix of standardised i4.0 data transmission and processing techniques that also utilise specialised artificial intelligence (AI) algorithms to turn that data into the means of implementing effective predictive maintenance regimes. “Sensor data gets sent to us in the cloud and then our proprietary AI algorithms can identify very subtle changes that enable us to tell the user exactly when a piece of equipment is going to fail,” explains Dr Kampa. Though the company is still relatively new and small it has taken on big projects; Senseye is already working with Nissan, Dr Kampa confirms, “monitoring 10,000 machines across seven factories” for the multinational OEM, though more specific details of that project remain confidential.
Dr Kampa notes that as a general rule, given that a single machine would have multiple sensors and that an individual sensor might monitor more than one parameter, the actual number of “data points” in an installation might well be as many as “fifteen times” the number of machines involved. He also adds that the company’s cloud computing resources necessarily process a “huge amount of data”.
The aim of all this, Dr Kampa says, is to enable predictive maintenance to be carried out “at scale”, something just not feasible if the data gathering is carried out by means of “handheld devices” and the data processing by “individual engineers”. He also reiterates a point that is often made – that much of the necessary data is already gathered by existing sensors on shopfloor equipment. “There is usually enough data on a shopfloor to get you started, though once you have proved the concept you might want to retrofit more sensors” he confirms.
Instead, states Dr Kampa, the “biggest challenge” tends to be “getting the data to the cloud.” As he explains, apart from a likely requirement for some enhanced shopfloor networking capabilities there may be more strategic security-related issues resulting from the fact that “the shopfloor network will usually be physically disconnected from the enterprise network that will be connected to the internet.” That will, he adds, normally involve first loading and then extracting the data to and from an existing system on the enterprise network that might, for instance, be a commercially available ERP product but could also be an in-house development.
But once the data is uploaded so that the “machine learning” embodied in the system can begin to analyse it and recognise any anomalies then, says Dr Kampa, just “14 days are required before the system can return value to users.” The interface by which it communicates predictive information back to users is, a “dynamic website” that can be viewed in numerous ways including, if required, on a display screen on the shopfloor where the equipment being monitored is actually located. At a microscopic level he says that if the data is sufficient then the system could accurately predict a failure “at the device level” as far as “six months in advance”.
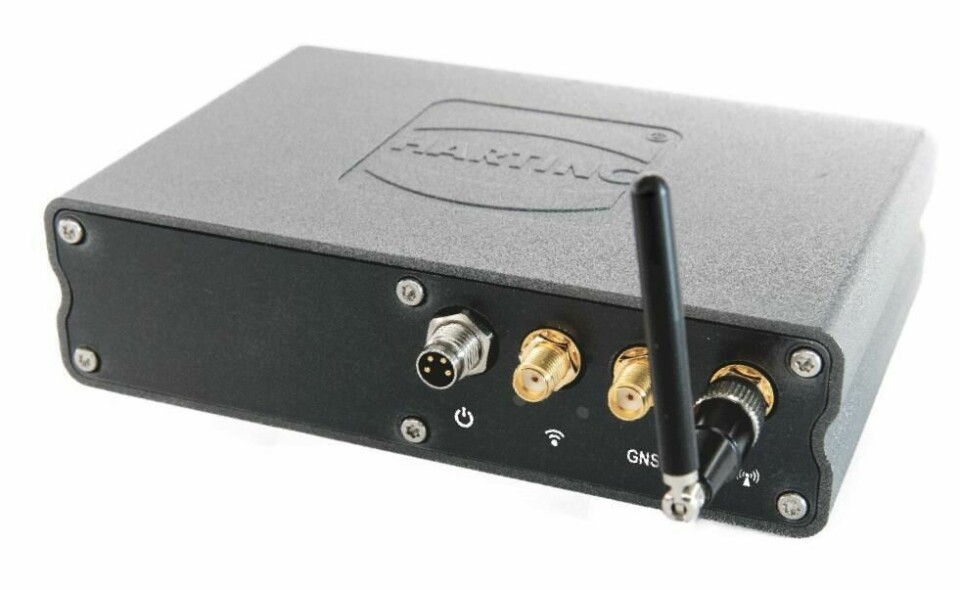
Conveyor condition monitoring
Meanwhile, a major automobile manufacturer in Germany has played a role in the development of a condition monitoring system that is now being used for the early detection of wear in the overhead conveyor systems typically used in production lines in the automotive industry. The system utilises the MICA (modular industry computer architecture) Edge computer system technology from supplier Harting to help avert the failure of individual support frames in these conveyors, which can lead to the stoppage of entire production lines. The essential capability of edge computing is that it makes it possible temporarily to save, evaluate and process data in the immediate vicinity of machinery and equipment.
Dr Jan Regtmeier, director of product management for Harting IT software development, based in Espelkamp, Germany, says worn rollers or damage to drives can lead to the failure of individual support frames, which inevitably leads to a bottleneck of the entire production line due to the serial guidance of an overhead conveyor. But the new monitoring system enables digital condition monitoring of the supporting frames so that creeping wear can be detected, and a warning of a potential failure issued in advance. The goal is to achieve higher overall equipment effectiveness (OEE) of the suspended conveyor.
The system consists of two sensor boxes and a MICA Edge computer, which directly evaluates the sensor values and transmits them to the control system via an integrated Wi-Fi function. The sensors continuously detect vibrations on the rotors, the frequency of the motor current, the motor current and the temperature of the drives. If a threshold value is exceeded the moving-state monitoring set sends a message to the operating equipment via the control system. Affected supporting racks can then be taken out of service in a targeted manner and serviced.
Data reduction and transformation
“This application is mainly about data reduction and data transformation,” explains Dr Regtmeier. “The data is sent via Wi-Fi to the cloud. But, given that every cloud requires a specific data format and authentication, this is effected by the edge capability so that the data can then be further processed in the cloud.” The condition monitoring set can be retrofitted for all types of transport systems with slow moving components, such as escalators, elevators and conveyor belts. Thanks to the IP 65/67 protection class of the sensor boxes and the MICA, the system can also be operated without problem in numerous ambient conditions.
“The sensors are optimised for slow moving rotating objects with high sensitivity even in a rough and shaking environment,” confirms Dr Regtmeier. “The data is already pre-processed on the sensor itself.” As such, says Dr Regtmeier, the system can be the basis for predictive analytics. “It combines a ‘smart IIoT sensor’ with edge computing and cloud data analysis,” he states. He also confirms the importance of the cooperation with a real manufacturing operation for the successful development of the system. “This is the best way to realise i4.0 applications,” he states.